Using Bollinger Bands, we can create a trading strategy to buy and sell stocks based on the market’s moving volatility.
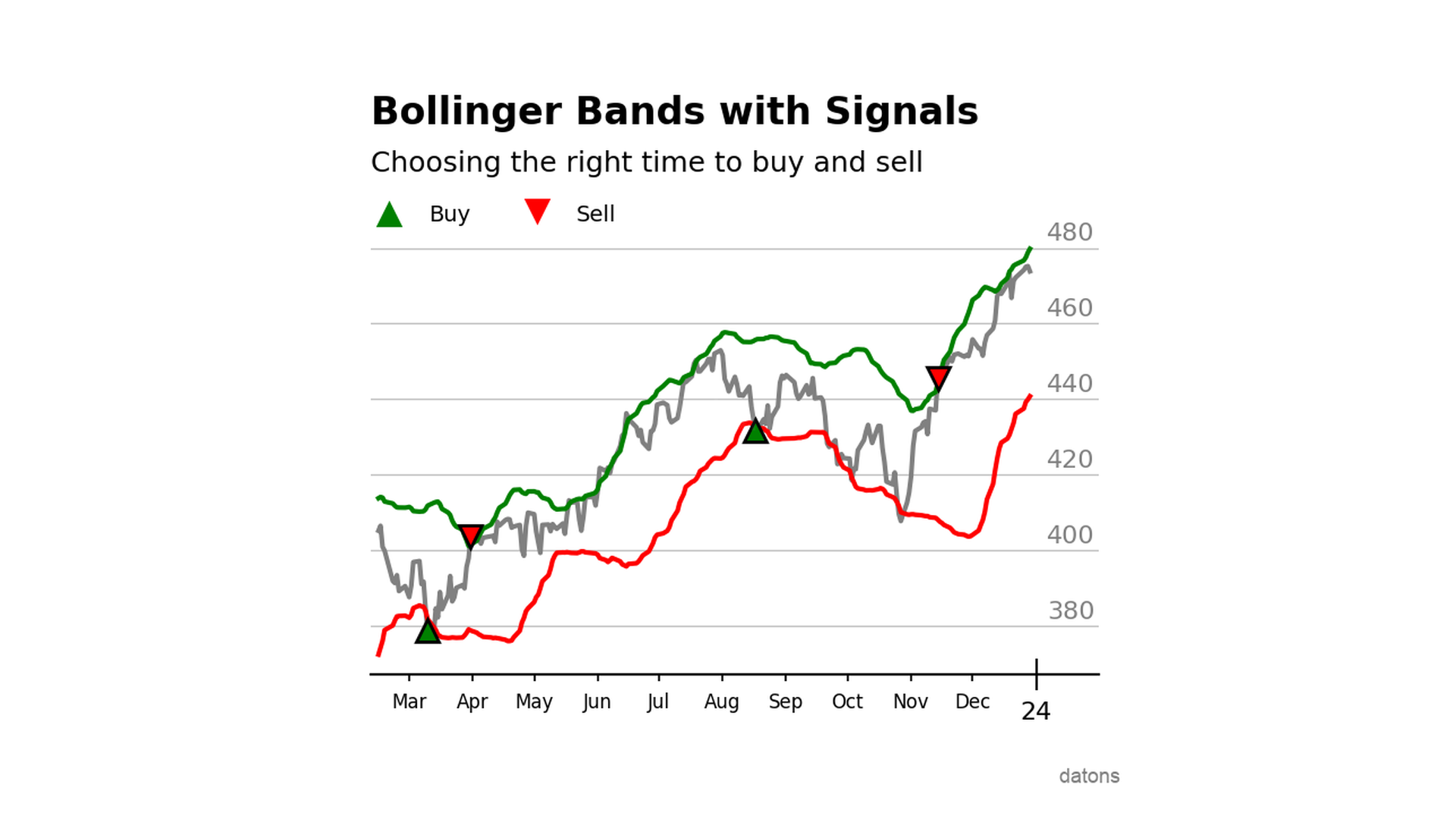
We explain the process as we apply the strategy to the S&P500 data.
Data
The S&P500 is an index that represents the performance of the 500 largest companies in the United States.
To simplify understanding, we selected one year of data.
import yfinance as yf
df = yf.download('SPY', start='2023-01-01', end='2024-01-01')
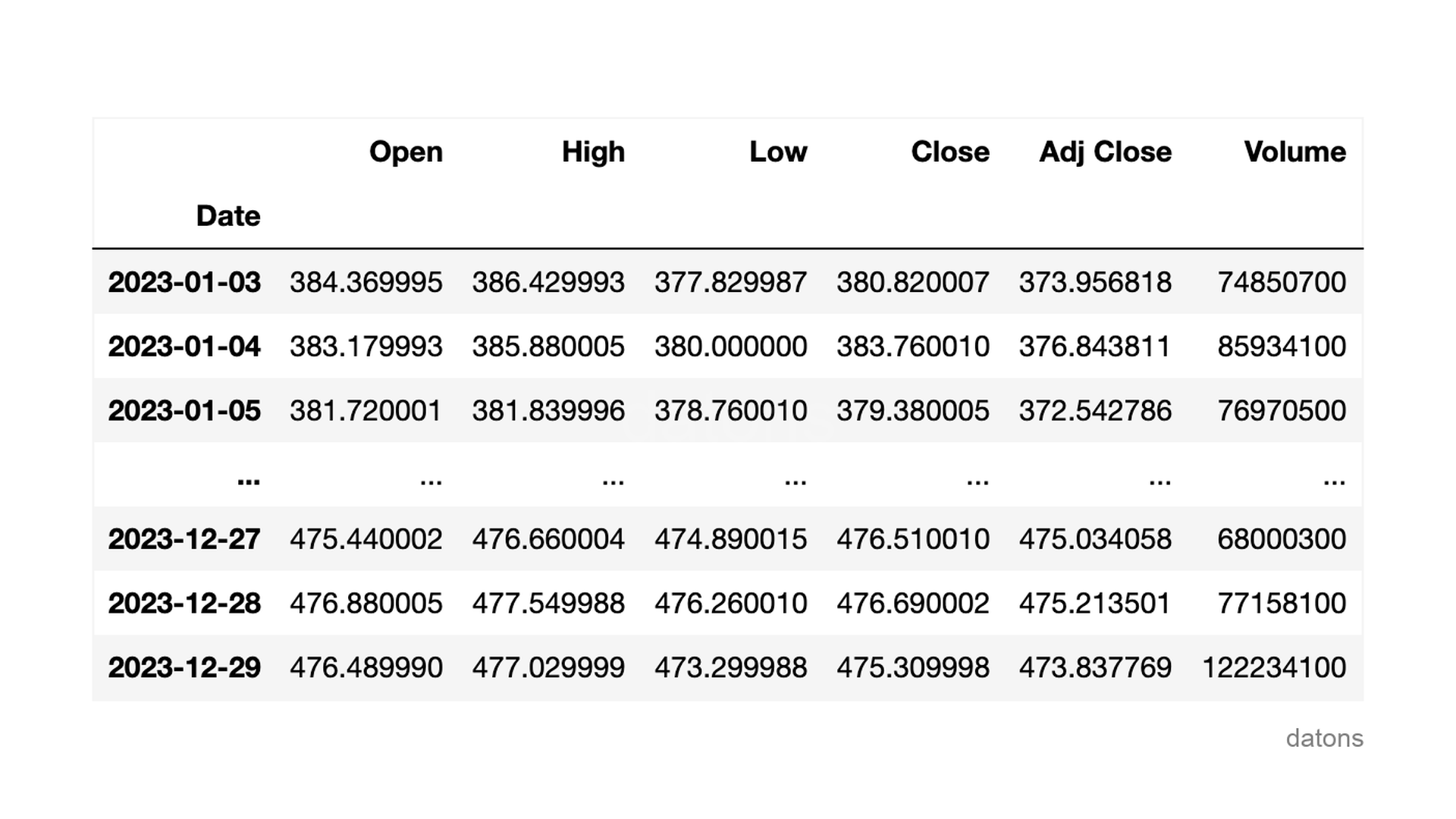
Questions
- What are Bollinger Bands, and how are they calculated?
- How to determine buy and sell signals with Bollinger Bands?
- How to avoid consecutive buy or sell signals in the trading strategy?
- How to calculate the outcome of the strategy?