Based on the energy generation bids recorded by EOLICA AUDAX
(ADXVD04)
in the OMIE market during 2023, this article
presents an analysis of anomalies in the time series.
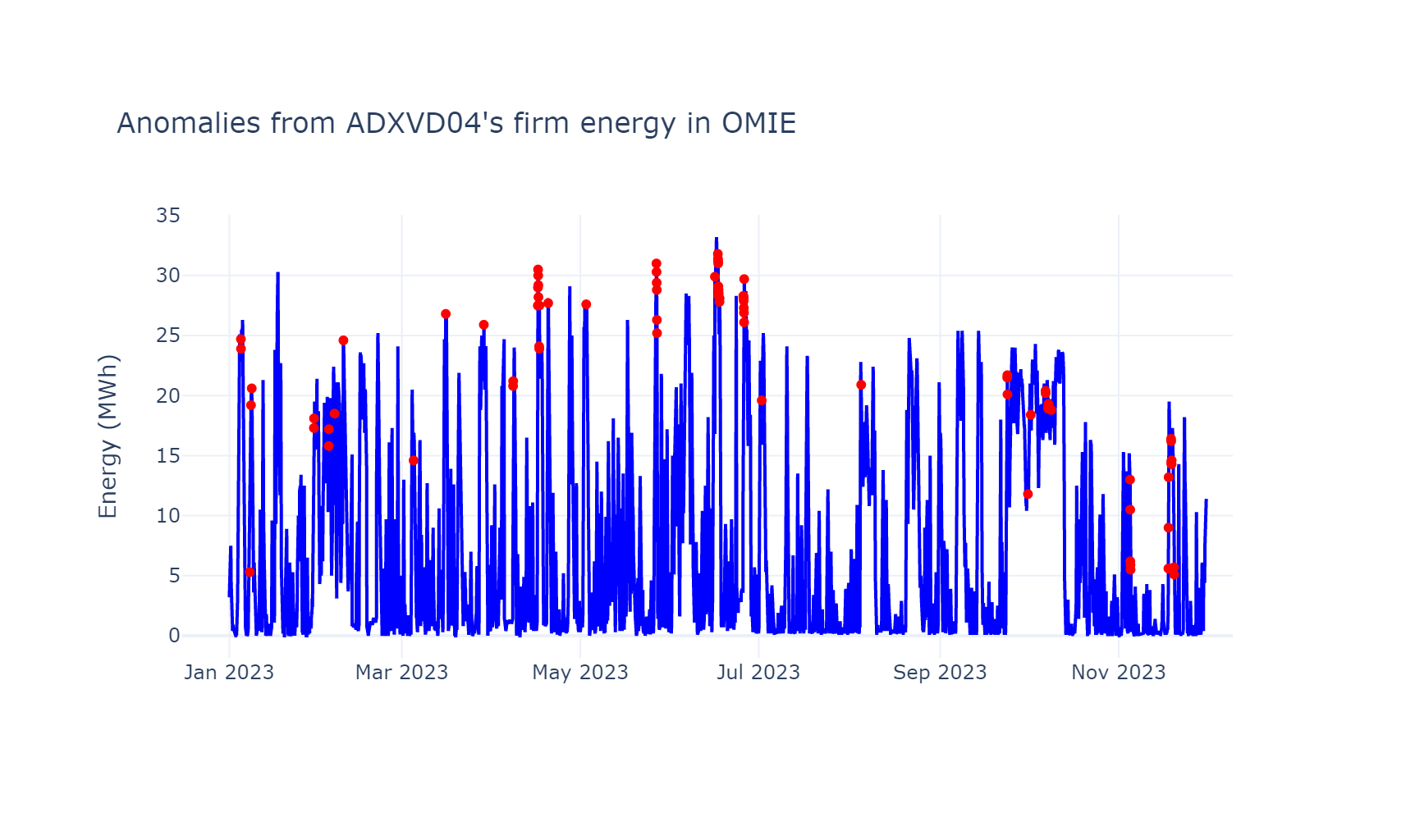
In this tutorial, you’ll learn how to develop an anomaly detection model for time series with Python based on a practical case.
Data
Each row represents the energy that the bidding unit
ADXVD04
has recorded in the OMIE market during 2023.
import pandas as pd
df = pd.read_csv('data.csv')
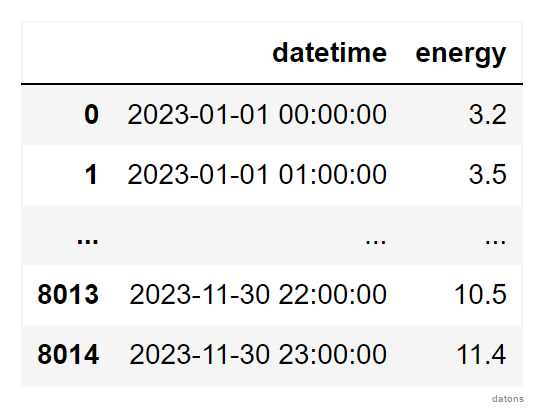
Questions
- How to extract temporal properties to detect anomalies?
- How to use the Isolation Forest algorithm to identify anomalous data?
- How to configure the algorithm to detect a specific percentage of data as anomalous?
- What techniques are used to visualize anomalous data in the time series?
Methodology
Temporal Columns
Following the steps of this tutorial, we create temporal columns that could explain the reason for anomalous data.
df.datetime = pd.to_datetime(df.datetime)
df.set_index('datetime', inplace=True)
df = (df
.assign(
month = lambda x: x.index.month,
hour = lambda x: x.index.hour,
)
)
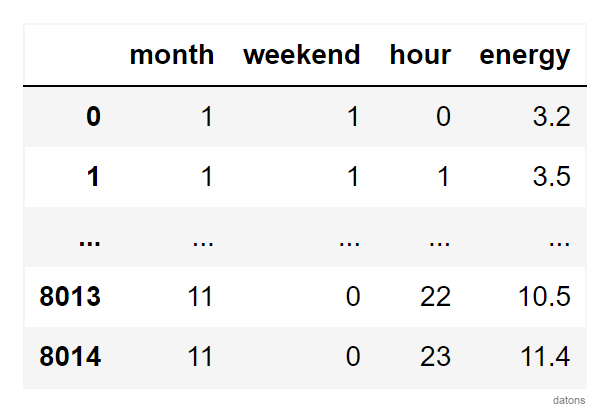
Anomaly Model
To detect anomalous data, we use the IsolationForest
algorithm from the sklearn
library. We set the
contamination
parameter to auto
so the model
automatically detects anomalous data.
from sklearn.ensemble import IsolationForest
model = IsolationForest(contamination='auto', random_state=42)
model.fit(df_model)
Automatic Anomaly Percentage
Using the mathematical equation optimized by the algorithm, we calculate the anomalous data to visualize the percentage of anomalies.
Would this be interesting to one of your friends? Share it with them.
df['anomaly'] = model.predict(df_model)
(df
.anomaly
.value_counts(normalize=True)
.rename(index={1: 'Normal', -1: 'Anomaly'})
.plot.pie()
)
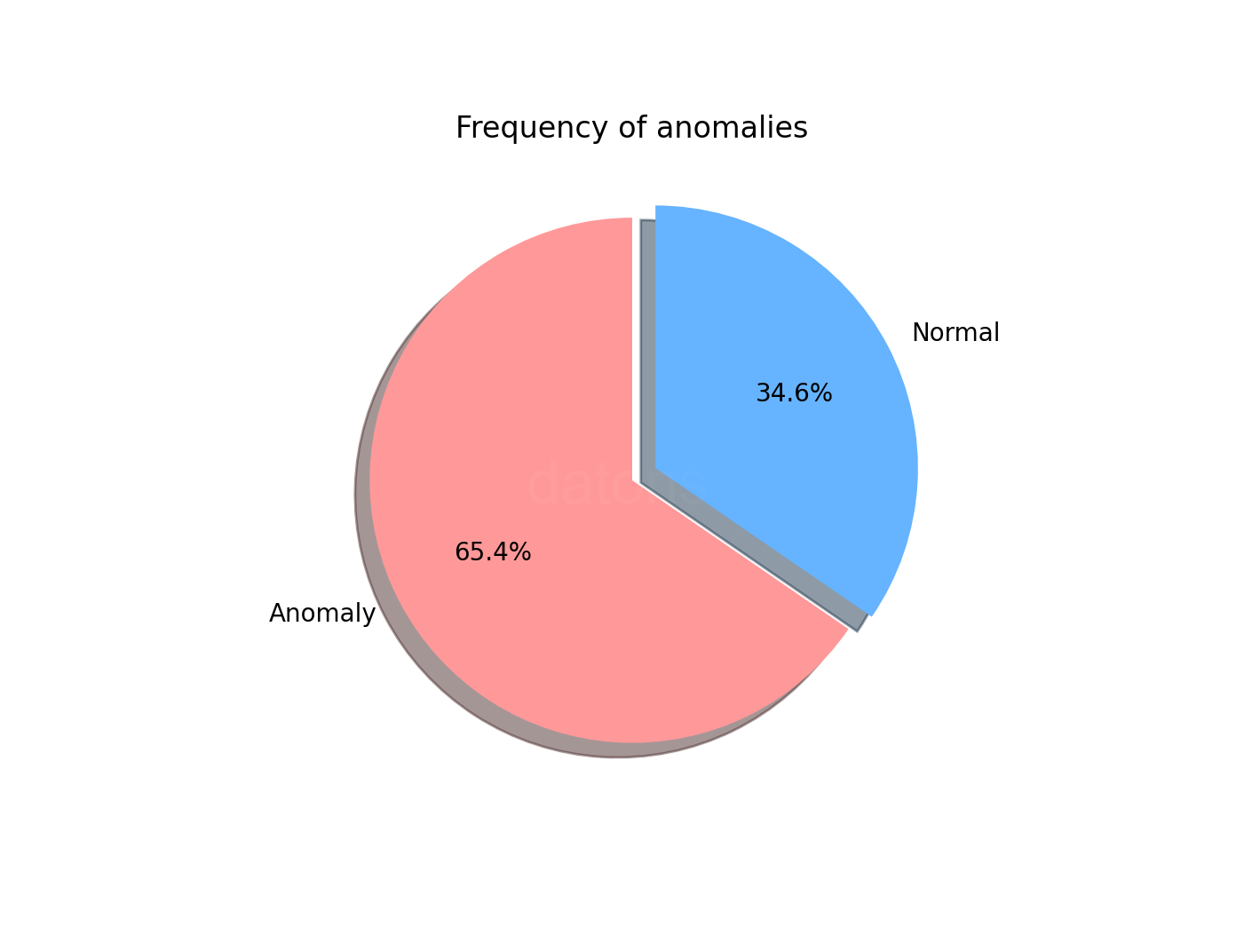
A 65.4% of ADXVD04
bids are anomalous according to the
model’s automatic setting.
Specifying Anomaly Percentage
It’s not logical for the majority of the data to be considered
anomalous. Therefore, we adjust the contamination
parameter
to 0.01
so the model detects 1% of the data as
anomalous.
model = IsolationForest(contamination=.01, random_state=42)
model.fit(df_model)
df['anomaly'] = model.predict(df_model)
Visualizing Time Series with Anomalies
Finally, we select the anomalous data:
s_anomaly = df.query('anomaly == -1').energy
And visualize them with points on the original time series using the
graph_objects
sublibrary of plotly
.
import plotly.graph_objects as go
go.Figure(
data=[
go.Scatter(x=s_anomaly.index, y=s_anomaly, mode='markers'),
go.Scatter(x=df.index, y=df.energy, mode='lines')
]
)
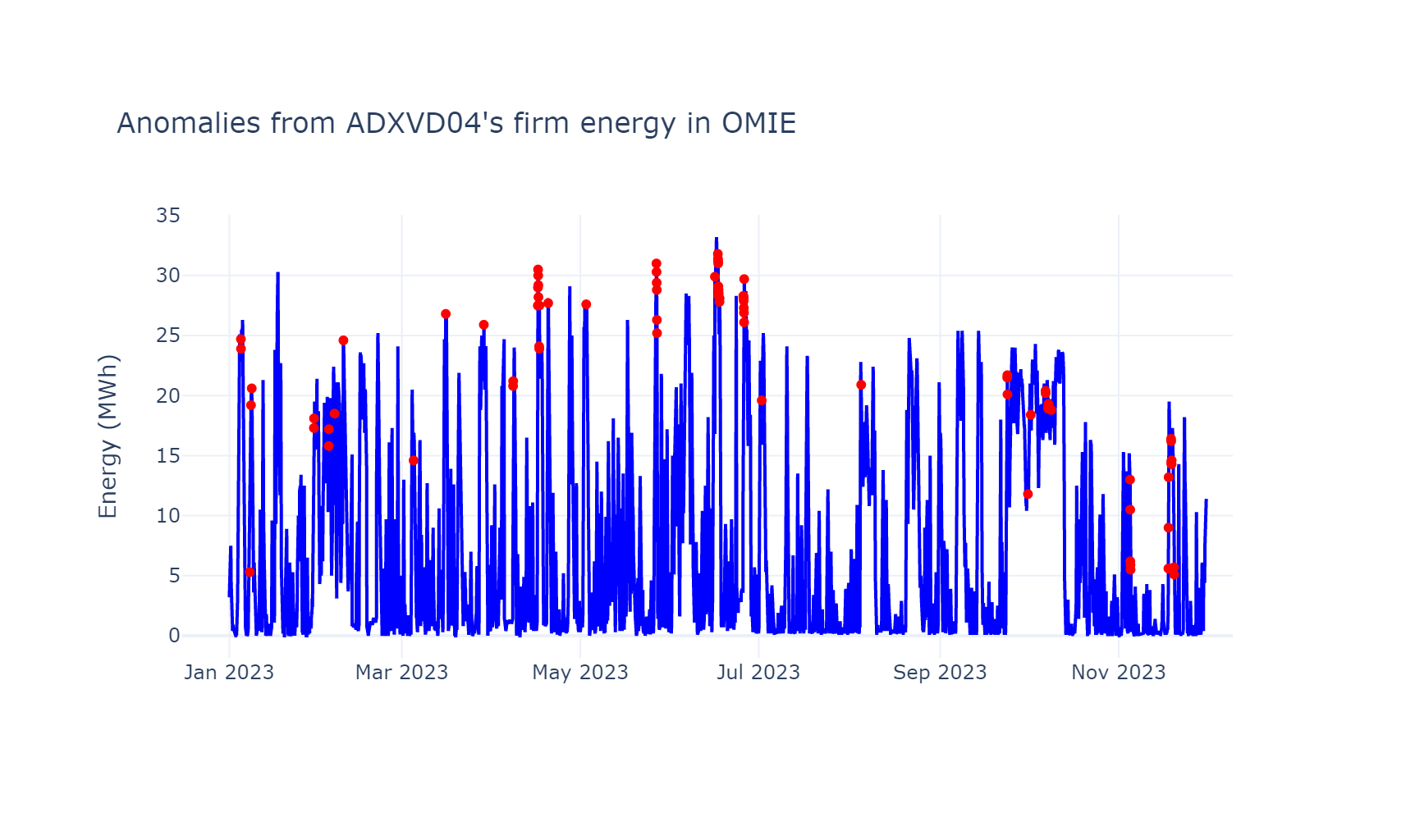
We can observe that the model detects anomalous observations, especially in the peaks of the time series.
What else could we do to analyze the anomalies? I’m looking forward to your comments.
Conclusions
- Extraction of Temporal Properties:
df.assign
to createmonth
andhour
columns from theDatetimeIndex
. - IsolationForest Algorithm:
sklearn
includes this algorithm in its Machine Learning framework. - Model Adjustment for Specific Anomaly Percentage:
IsolationForest(contamination=0.01)
adjusts the model’s sensitivity to identify 1% of the data as anomalous. - Techniques for Visualizing Anomalous Data:
plotly.graph_objects.Figure
allows us to combine in a visualization both the anomalous data and the original time series.
If you could program whatever you wanted, what would it be?
I might give you a hand by creating tutorials that help you. I’ll read you in the comments.